Understanding the language of proteins
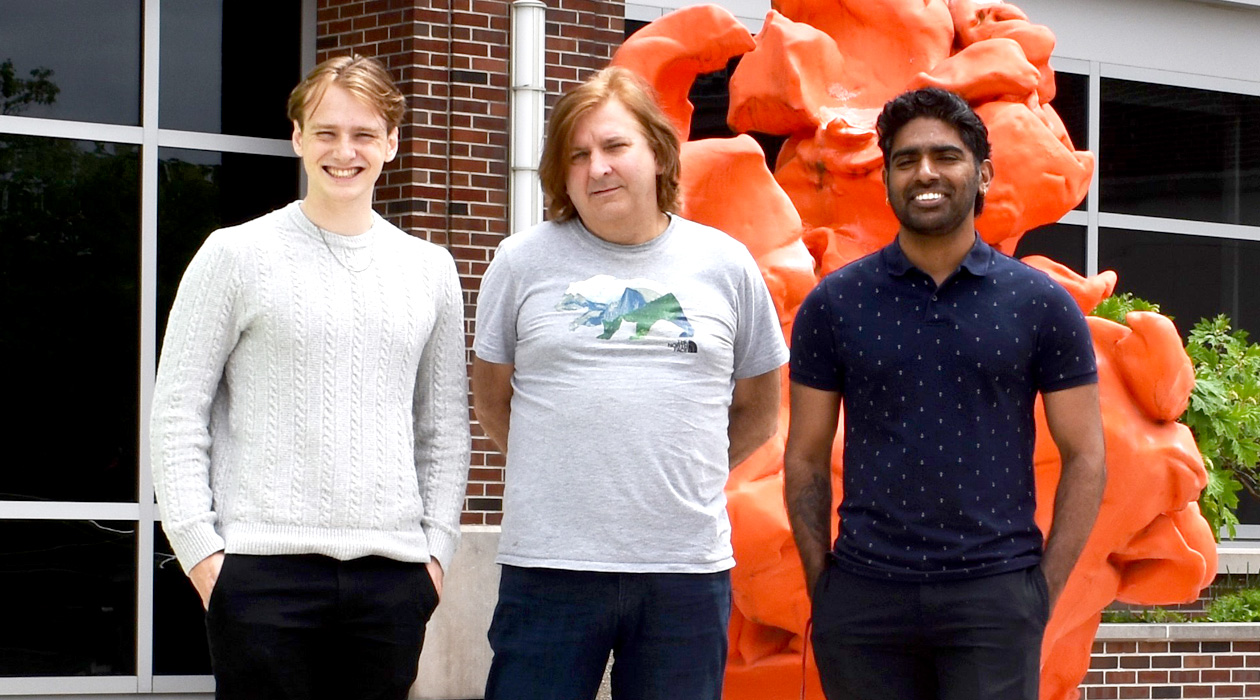
From left to right, John Malcolm Forsyth, Professor Sergei Maslov, Ananthan Nambiar / Stuart Robbins
Architecturally, Large Language Models are the basis for Generative AI popularized by ChatGPT from OpenAI, and similar products from Meta, Google, Microsoft, and C3.AI. By focusing upon specific tasks, AI algorithms are capable of faster responses to questions, expressed in natural language that we can understand.
Here at the University of Illinois Urbana-Champaign, there is an inter-departmental team doing exactly that – focused upon the “language” of proteins.
In their newly published essay (Structure, Cell.com, May 2), co-authors Ananthan Nambiar, John Malcolm Forsyth, Simon Liu, and professor of bioengineering and Bliss Faculty Scholar Sergei Maslov (CAIM co-leader/CABBI) have developed a protein language model focused upon understanding and predicting the behavior of disorganized regions of proteins. In the introduction to their paper, they explain that the core idea of protein language modeling is that amino acids that comprise a protein are analogous to words that comprise a sentence.
In a recent interview, the team was asked what distinguishes their efforts from those mentioned above.
“Dr. BERT runs on a laptop,” according to Professor Maslov.
“...with no GPUs, and it was trained with 2 Nvidia GPUs...” Ananthan Nambiar added with a smile. (Meta’s infrastructure, for example, involves more than one hundred Nvidia chips.)
The team accomplished remarkable performance improvements when compared to similar models for predictive analytics for proteins. With fewer parameters and minimal data pre-processing, DR-BERT is more accessible to users/researchers who may not have access to high performance infrastructure.
“Dr. BERT” is a nickname for DR (Disorganized Regions) and BERT (Bidirectional Encoder Representations from Transformers), a new protein language model for predicting disordered regions in proteins. It promises a significant contribution to protein language modeling, a fast-growing area of deep learning research for computational biology with increasing interest and investment throughout the BioTech industry.
Whether the teams are large or small, language modeling involves heightened attention to pre-training of their models. DR-BERT was pre-trained on ~6 million unannotated protein sequences, then fine-tuned to predict “disordered regions” utilizing a much smaller dataset. In their research, validated by extensive comparative testing, they have been able to demonstrate that, despite its compact nature, DR-BERT performed competitively with, and often surpassed by, the accuracy of existing models.
“This is really Part 2,” Ananthan explained. Based upon their earlier research published by the Association of Computing Machinery (Proceedings of the 11th ACM International Conference on Bioinformatics, Computational Biology and Health Informatics, September 2020), the team is poised to continue refining the tools and results in the future.
One of the factors that contributed to the team’s work resulting in publication is rooted in the values of inter-departmental collaboration at the University. Professor Maslov is convinced they are positioned for continued cross-department success, “particularly with the surprising contributions of our undergraduate colleague, Malcolm…”
What’s comes next for the DR-BERT team?
Ananthan believes there are broader benefits. As noted in their paper (see link below), “…the success of DR-BERT, in addition to the insight into how DR-BERT makes predictions, leads us to believe that protein language models could play an important role in the next generation of neural networks…”
For the full text of their recently published article, see DR-BERT: A Protein Language Model to Annotate Disordered Regions.
Authors
Professor Sergei Maslov (University of Illinois Urbana-Champaign Department of Bioengineering, Center for Artificial Intelligence and Modeling, Carl R. Woese Institute for Genomic Biology, Department of Physics, NCSA, and Computing, Environment and Life Sciences, Argonne National Laboratory). Ananthan Nambiar (Graduate Researcher, Department of Bioengineering, Carl R. Woese Institute for Genomic Biology); John Malcolm Forsyth (Undergraduate Researcher, Department of Computer Science, UIUC); Not pictured: Simon Liu (Department of Computer Science, UIUC, currently at Roblox, Inc., in San Jose, CA).
Acknowledgements
This team’s work utilized resources supported by the National Science Foundation’s Major Research Instrumentation program, grant # 1725729, and part of their work was performed under the auspices of the U.S. Department of Entergy by Argonne National Laboratory, under contract DE-AC02-06-CH11357.